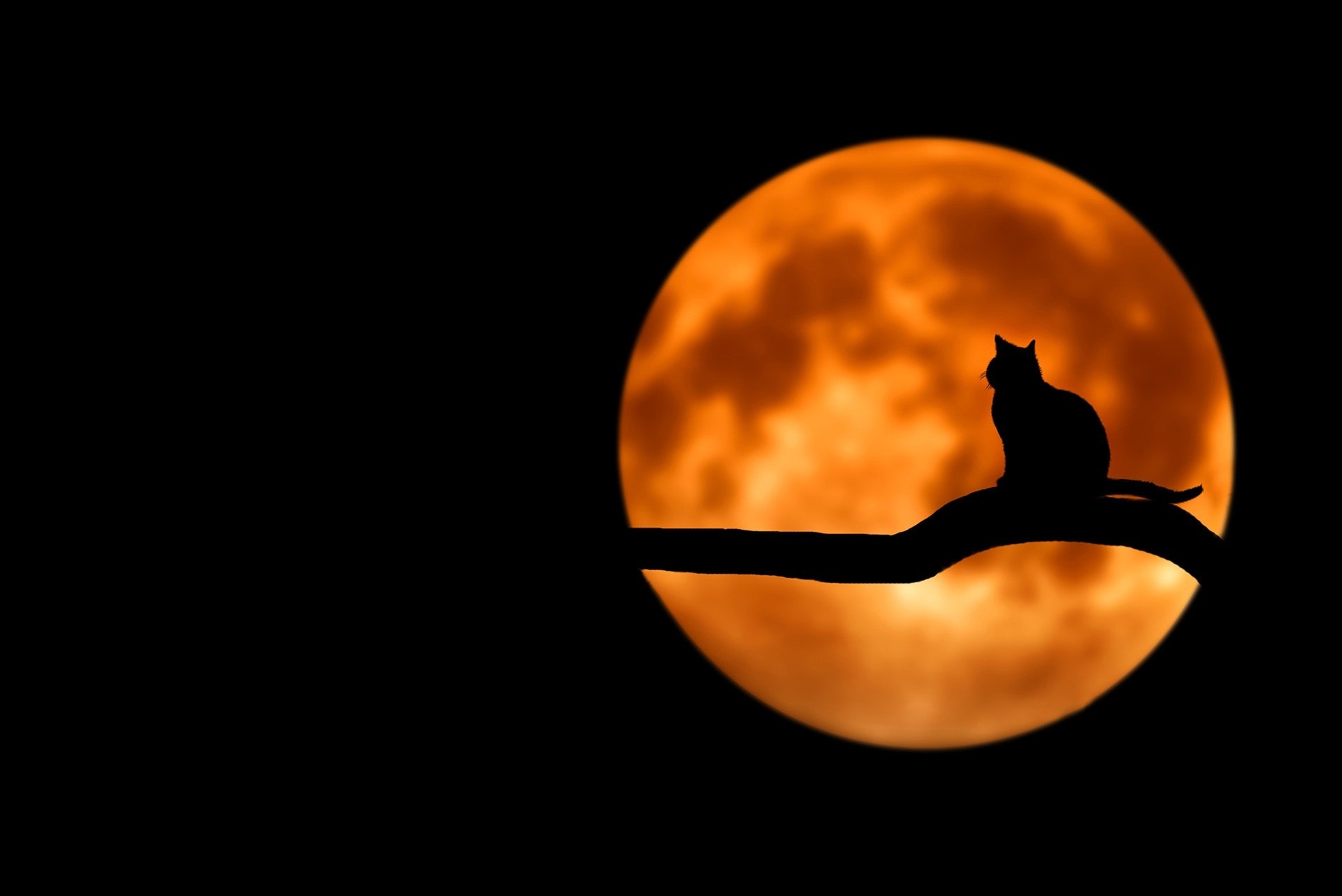
animal-branch-cat-35888
AIDA blog: AI’s black box: Trick or treat?
Halloween times are upon us. You may or may not embrace this tradition, but you probably won’t be able to escape this celebration of fright. Of course, it made me relate to the AI domain (it’s an occupational hazard) and the horrors we find there.
What many would consider the scariest thing with AI is its black box characteristic. Exactly how a deep learning model makes its prediction cannot be explained by understandable means such as a set of distinct criteria. Displaying a network with all its weights doesn’t help much either. For some, this is a fundamental reason to distrust AI. In their mind, an AI solution poses a ‘trick or treat?’ question, but with the difference that you’re not making the choice – it will silently be made for you.
Myself, I’m not scared. These concerns are valid, and care must be taken to avoid pitfalls, but there are several reasons why the anxiety can be kept at bay.
First of all, I’d like to question whether AI is much different than other tech in this respect. Compare to a computed tomography (CT) scanner, typically not seen as a black box system. I imagine that there are, indeed, detailed specifications of each part of the system. Yet, the inner workings of such a complicated amalgamation of technical components is by no means easy to grasp. In fact, I would challenge anyone to describe the complete provenance of a pixel value in the image data resulting from a CT scan. Even though it may be theoretically possible to inspect the full pipeline at full granularity, it’s probably impossible in practice, and it’s definitely unfeasible to do so. To me, that sounds like a black box.
We must also consider the typical alternative: human expertise. Now there’s a black box. While radiologists and pathologists to some extent can describe distinct findings supporting a decision, I’m sure they all would agree that for a complex case there is much more behind a diagnostic conclusion than can be captured in unambiguous semantics.
Still, both CTs and human experts can perform very valuable tasks with high quality. Why does it work? Because we do quality assurance. We test and test again to make sure we get sufficiently correct and reproducible results. And this is going to be even more important when we deploy AI in clinical practice. Of course, testing during product development is crucial, but also when in clinical use. Just as we have hospital physicists regularly monitoring med tech equipment to check that it performs as it should, we must have hospital computer scientists monitoring AI performance. Is the model as precise when a new CT or new histology staining system is installed, or as the patient characteristics change over time?
My second point is that the blackness of the box is often exaggerated. The research field of explainable AI (XAI) has already delivered many useful ways to interpret the underpinnings of a prediction, and much more is to come. As an example, many AI errors can be spotted by highlighting the area in the image being most important for the prediction.
Thirdly, there are scenarios when black box methods are to be embraced even if they are completely obscure. In an opinion piece in Science (http://science.sciencemag.org/content/364/6435/26), Elizabeth Holm argues that there are three main reasons to adopt black box methods: when they produce the best results, when the cost of a wrong answer is low, or when they inspire new ideas. I concur, also with her caution that any black box must be used with knowledge, judgment, and responsibility.
Perhaps chances are slim that a black-box-AI monster will be among the creatures haunting your neighborhood this Halloween. But whenever you see one, my advice is that you try to unveil it. Studying it in bright daylight will make it a lot less scary.

AKTUELLT
Ny rapport visar hur viktigt medicinteknik är för Sverige
En ny rapport från Vinnova visar hur viktigt medicinteknik är för Sverige. En årlig kartläggning [...]
Nu öppnar Medtech4Health Innovation Award 2025
Nu öppnar nomineringen till 2025 års Medtech4Health Innovation Award – priset som belönar innovativ medicinteknik. Nomineringen öppnar den 16 oktober och stänger den 1 december.
Medicinteknikdagarna i backspegeln
Årets Medicinteknikdagar bjöd på otroligt spännande sessioner allt från forskningsnära nya teknologier, insikter om möjligheter [...]
Vinsten förstärkte Mindmores verksamhet och teamkänsla
Medtech4Health Innovation Award 2024 gick till Mindmore – ett digitalt verktyg för bland annat kognitiv svikt. Att valet föll på Mindmore berodde även på att företaget har lyckats väl med en bred implementering: Mindmore används idag aktivt av både regioner och privata vårdgivare .
NYHETSBREV
Följ nyheter och utlysningar från Medtech4Health - prenumera på vårt nyhetsbrev.