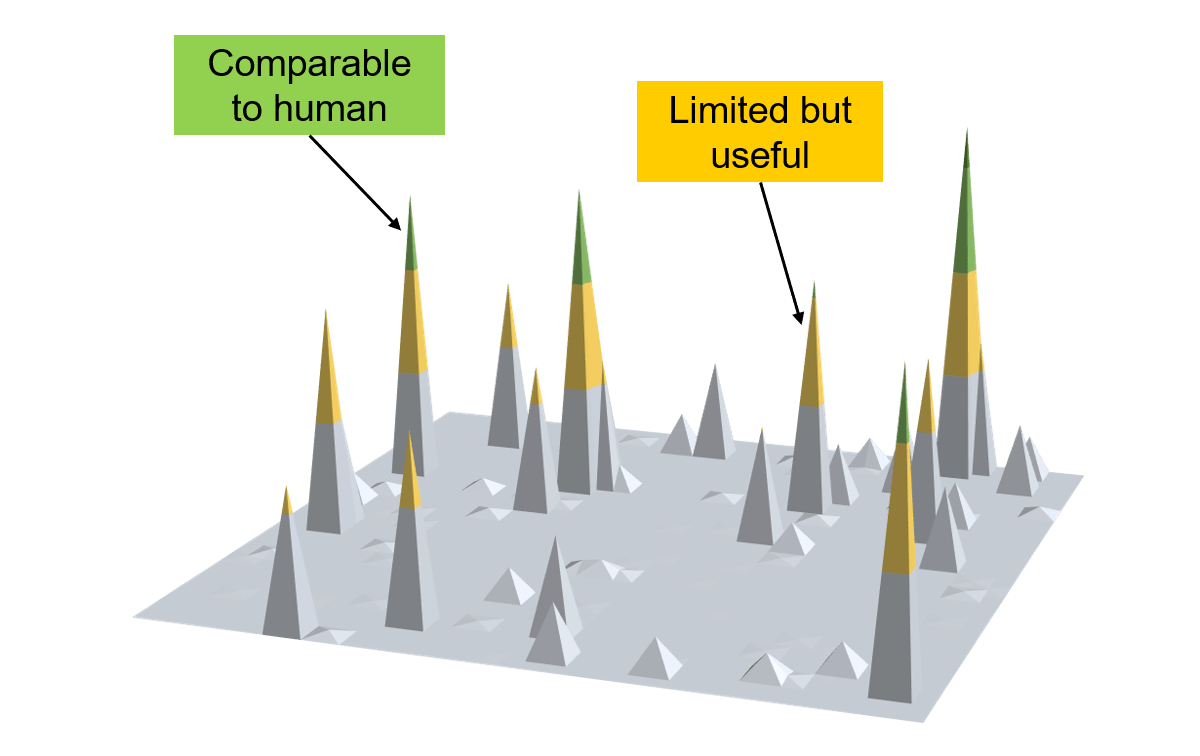
AI landscape
AIDA blog: AI is as good as radiologists… and nowhere close
Part of my job is to spread knowledge on AI, foremost with respect to imaging diagnostics, to audiences with limited experience in the domain. From those interactions I’ve learnt that one issue is particularly puzzling if you’re not actively working in the area. On one hand, there are frequent reports of the type “the machine is better than the human experts”, the experts typically being radiologists or pathologists when related to AIDA’s operations. On the other hand, the accounts from the clinical reality is that the usage of modern AI is extremely scarce.
The two views seem to be mutually exclusive. It may be tempting to simplify to a black-and-white question, select one of the trenches and start arguing for that people in the other trench is wrong. But my take on it is that both views are correct. The figure above is an illustration that I’ve found to be very helpful in explaining this pseudo-paradox.
Consider all the explicit and implicit tasks and assessments that a radiologist or pathologist does during diagnostic work, and picture it as a landscape. Now let the current capacity of AI solutions to perform a subtask be represented by height in this landscape. At the highest level, AI is as good as the diagnostician.
What we then see is indeed the high peaks, here color-coded in green, where AI has been proven in experiments to match or surpass humans. But we also see that the landscape is extremely scattered. The peaks are yet few, and very far apart. And they are very narrow. I think there is a common misconception that a high-performing AI model also is high-performing in generalizability. But as we know, the typical reality is that performance degrades dramatically outside the original training sandbox.
Despite the top capacity, the current very limited coverage means that it is unreasonable for AI to broadly replace human diagnosticians anytime soon. This was the topic of an April Fools’ Day prank earlier this year, where Hugh Harvey tweeted about a full radiology department being replaced . I don’t think anyone working in the domain was fooled, but I imagine many others were.
The key to making use of AI is to harness the amazing capacity of the peaks, while carefully integrating them in a clinical workflow that covers the broader landscape. In some cases with very well-defined independent subtasks, AI solutions can probably quite soon work with a fair degree of independence, with human experts having more of a quality assurance role. As many others, I think screening applications may be first in line. But one should acknowledge that AI solutions far below human expertise level can also lead to great benefits as accelerators for human diagnostic work.
I see the development of AI in our domain as populating this landscape with more and more peaks, and raising the height of the existing peaks. This is a bed of nails to look forward to, it can really transform healthcare for the better. No matter how dense the landscape is, however, I expect that carefully designed clinical workflows connecting the pathways in this landscape will remain the key to successful AI adoption.
Footnote: I’d say that the landscape metaphor could work on several levels – when encompassing all radiologists’/pathologists’ work, all work of a single radiologist/pathologist, and in most cases all radiologist/pathologist work for a single case. Pick the one that suits your rhetoric.

AKTUELLT
Delta med oss på Framtidens Hälso- och sjukvård i Stockholm
Medtech4Health finns på plats med monter och dubbla seminarium under Framtidens Hälso- och sjukvård den 22-23 januari 2025. Som medlem i Medtech4Health-familjen finns möjlighet att ta del av ett rabatterat erbjudande till konferensen.
Två nya utlysningar öppnar i januari 2025
I januari öppnar utlysningarna IMPlementering av medicinteknik inom vård och omsorg och Kompetensförstärkning i småföretag.
Game Changing MedTech – nya medicintekniska lösningar som verkligen gör skillnad
Apotekarsocietetens årliga heldag om medicinteknik gick av stapeln 6 december. Temat var banbrytande lösningar för framtidens vård. Dagen rymde allt från innovativa exempel ur dagens vård till framtidsspaningar.
Medtech Morning om framtidens vård och ersättningsmodeller
Under årets sista Medtech Morning i december samlades ett 50-tal deltagare kring frågan: hur kan vi främja fler medicintekniska innovationer i vården och hur ser framtidens ersättningsmodeller ut?
NYHETSBREV
Följ nyheter och utlysningar från Medtech4Health - prenumera på vårt nyhetsbrev.